Research Interest
Computer Vision
Image and Video Restoration
Underwater Vision
Segmentation of Aerial Images
Image Memorability
Robotic Vision
Zero Shot Learning
Active Learning
Medical Imaging
Human Gait Analysis
Detection and Rehabilitation for Osteoarthritis
Adversarial Perturbation
Adaptive Video Streaming
Media Forensics
Steganography and Steganalysis
Watermarking and Data Hiding
Major Research Areas
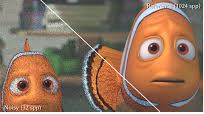
Observing the huge success of deep learning architecture such as convolution neural network in wide range of computer vision problems, recently, our group starts exploring different computer vision problems such as medical imaging, video saliency detection, media understanding, image and video steganalysis etc. using the deep neural network. See More
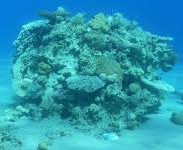
The problem of underwater image enhancement has been given considerable importance in recent times due to its vast application area in marine engineering and aquatic robotics. The two foremost reasons that make the underwater image restoration difficult are scattering and color distortion. See More
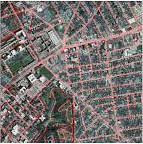
In MM lab we are focusing on various computer vision applications on aerial and satellite data with Deep Learning. This covers the aerial/satellite semantic segmentation, image translation, super-resolution, colorization, object Detection and tracking. See More
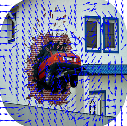
Image or object memorability is such a metric which describes a subjective property of an image. Latest research works show that it is not an incomprehensible concept: variation in remembering images is consistent across viewers. It suggests that independent of a viewers’ contexts and biases, some images are intrinsically more memorable than others. This research work proposes few visual factors which play a major role in determining memorability at object and image levels and few deep learning based memorability prediction models are proposed to predict object and image memorability scores individually. See More
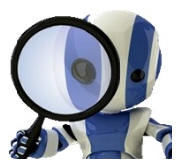
Robotic vision is concerned with acquiring visual information by a robot from its surroundings and using that as feedback to perform physical tasks. Leveraging general deep learning-based visual frameworks to perform such tasks in dynamic environments poses huge challenges. This is because these frameworks usually learn from datasets comprising a limited set of categories of objects, and in real-world scenarios, new objects may be encountered. See More
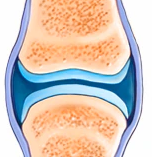
With a huge advancement in AI, machine learning, and medical assistive technology, the visual and bio-marker-based diagnostic methodology has become a prominent research area. In the multimedia lab, we are exploring different machine learning based applications for human gait analysis and detection and rehabilitation for Osteoarthritis. See More

In a world where deep neural networks (DNNs) are heavily relied upon for image classification and other tasks in Computer Vision, the discovery of a major weakness in the form of adversarial perturbations, which can easily fool the DNNs into confident misclassifications, poses a security threat and compromises with the applications based on the performance of DNNs. Hence, it becomes important to devise detection techniques to catch any adversarial attack, and then have proper measures taken to fix the targeted models. See More
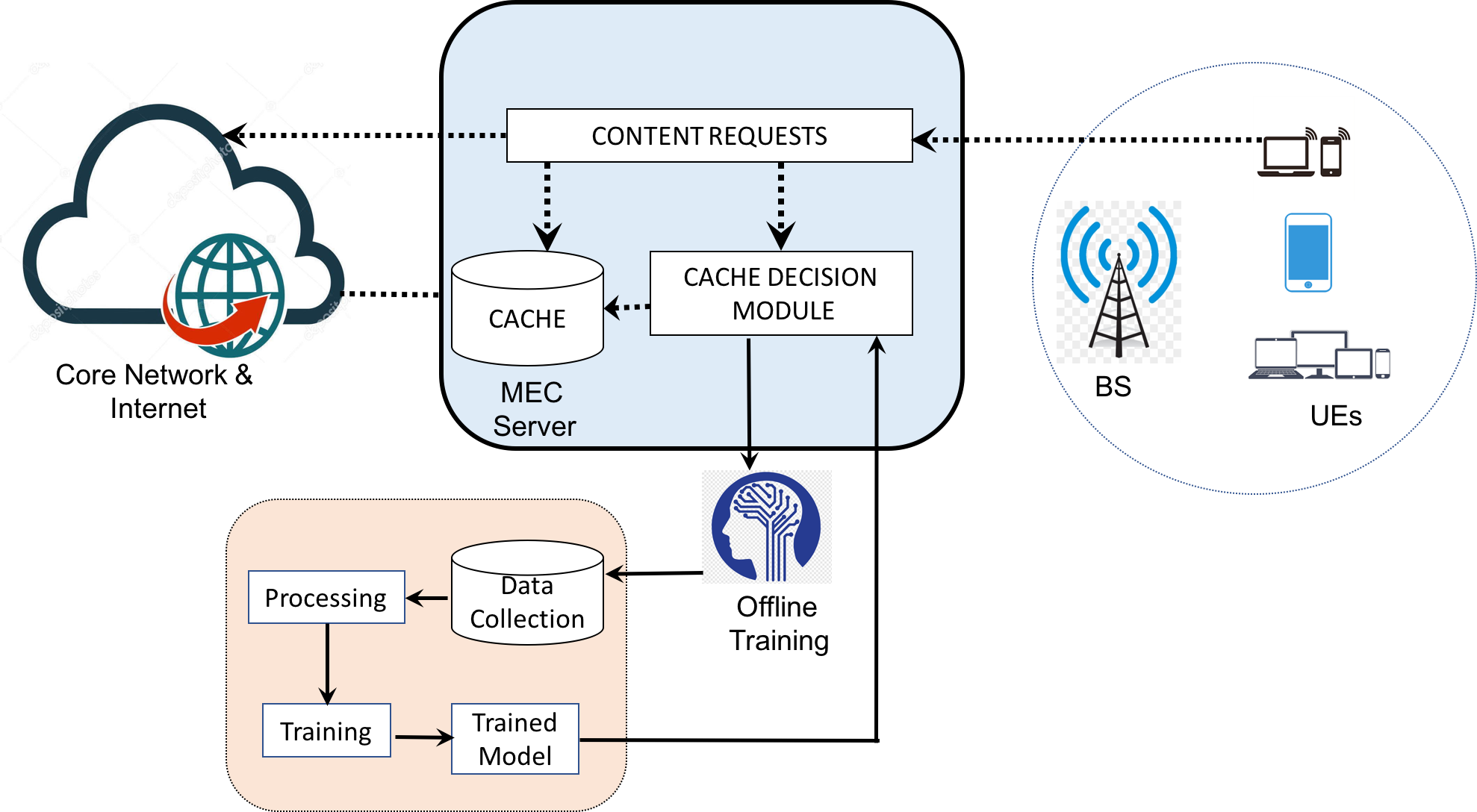
The integration of Mobile Edge Computing (MEC) into the cellular architecture, provides advantages like intelligent and efficient context aware caching, video adaptations for the delivery of content. In this work, the primary objective is to improve the video delivery to the heterogeneous end-users demands and varying network conditions. See More
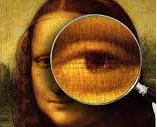
Steganography and steganalysis in digital images have been studied for decades. Recently, deep learning has been explored for steganography and steganalysis. The main goal of steganography and steganalysis using deep learning is to train deep learning-based models to efficiently learn to hide given secret information within the image and to detect the trace of the hidden information in the given image, respectively. See More