Research
Past Projects
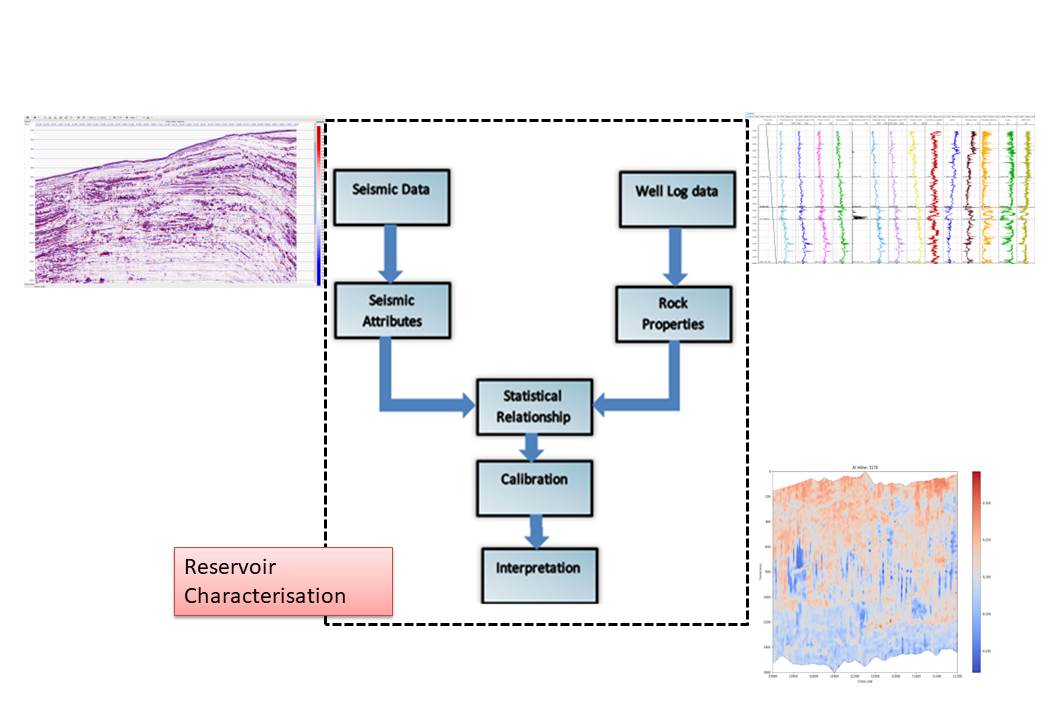
Estimation of Petrophysical Properties from Seismic Attributes and Well Logs Using Advanced Artificial Intelligence.
Funded by ONGC, Geodata Processing & Interpretation Centre (GEOPIC), Dehradun. Â
The success of oil exploration relies heavily on accurate reservoir characterization, which estimates the distribution of key petrophysical properties, such as porosity, sand fraction, and water saturation, from seismic signals. This task is challenging due to the complex and variable relationship between seismic signals and petrophysical properties. Our project aims to develop a user-friendly tool that leverages advanced AI techniques, including deep learning and ensemble methods, to provide accurate distributions of these properties across an oil field.Â
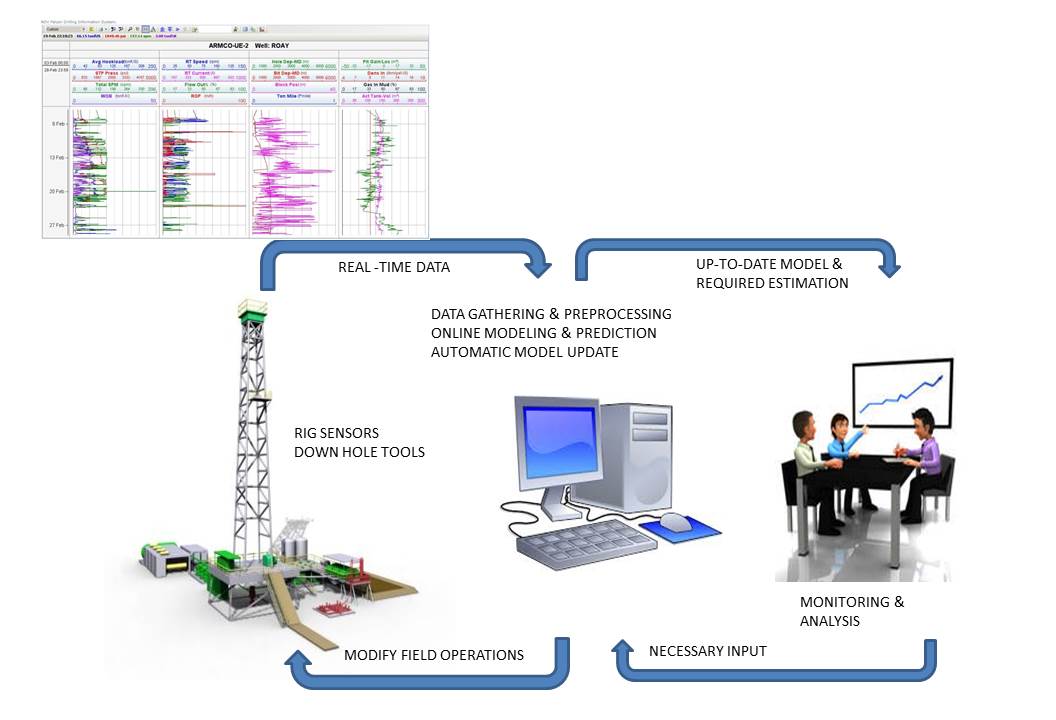
Development of Comprehensive Interactive Well Monitoring Software Tool for Drilling Supervisors
Funded by ONGC, Institute of Drilling Technology (IDT), Dehradun .
The objective of this project is to develop a real-time well drilling monitoring tool, which will make use of data from various sensors as well as data provided by the operators to enable advanced operation which includes: real time multi objective optimization (MOO) of drilling parameters to enable operators choosing feasible and optimal solution in real time, real time optimal preventive maintenance schedule for drilling units through machine learning techniques, fusion of MOO and machine learning techniques for complicated decisions, What-if analysis tool, real time energy and carbon audit for drilling operation to benchmark rig performance among others. This tool will help to improve overall performance of the well and reduce the Non-productive time thereby reducing the cost.
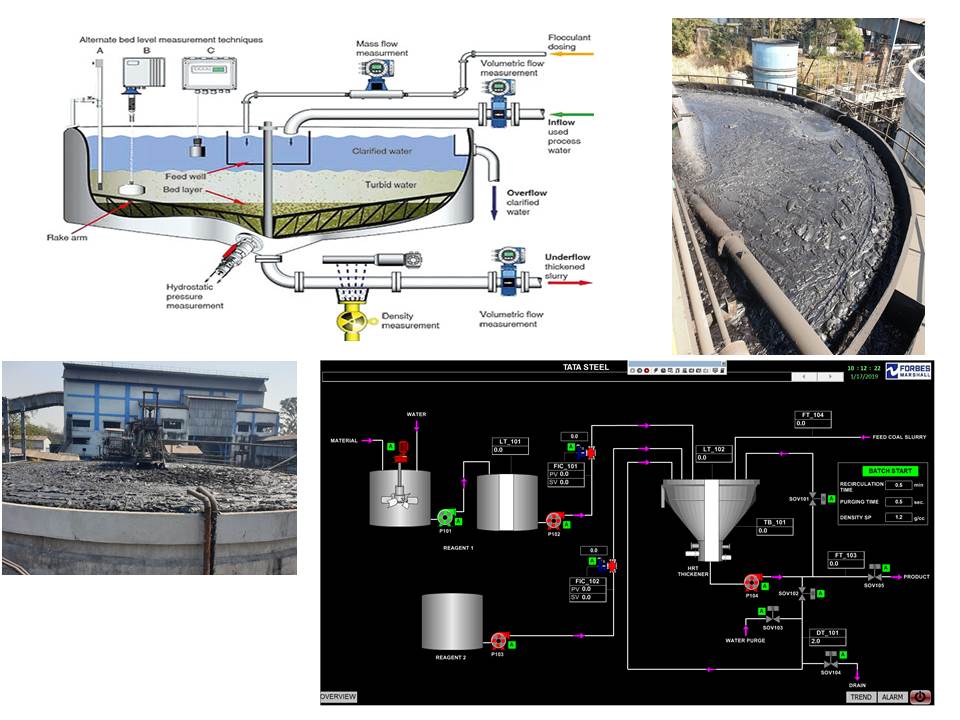
Estimation of flocculant dosage for Efficient Operation of High Rate Thickener (HRT) using Artificial Intelligence-based models
Funded by Tata Steel Limited, Jamshedpur.
Thickeners are equipment used for gravity separation of solids from suspension. The basic objectives are to increase the clarity of the overflow and to increase the solid particles concentration in the underflow. Flocculants are chemicals that help particles to agglomerate together to form larger size particles so that the particles settle faster. The flocculant dose is crucial to the thickener operation. The flocculant dosages are usually determined based on the settling rate studies conducted in the Lab. How- ever, focculant dosage will vary due to many factors such as variation in the feed to the thickener. Therefore, the dosage determined at the lab may not work for all the cases. The aim of this project is to develop AI-based models for estimation of flocculant dosage.
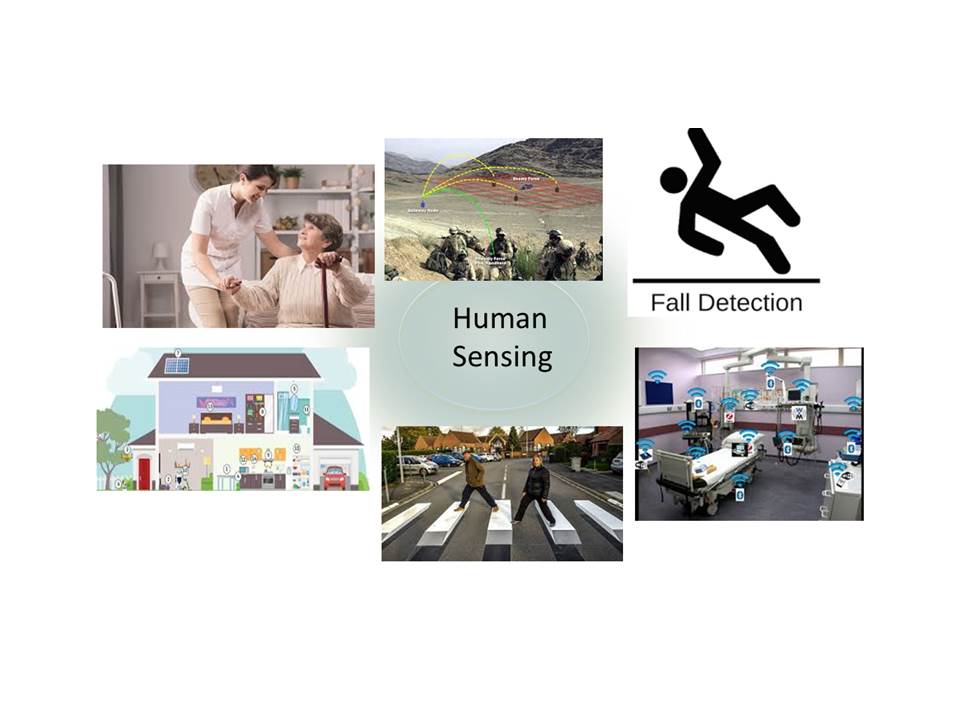
Non-Intrusive Human Sensing
Human sensing involves the detection of the presence of at least one human being in a given environment. It also pertains to the process of extracting information about the people in the given environment. Human sensing is required to ascertain the number of persons present in a room, to find whether a specific person is present within, where he/she was earlier, the path followed to reach the current location and maybe even identify a specific person among those present. To achieve this, researchers have investigated the use of multiple sensors coupled with different means of communication including Bluetooth, Wi-Fi, etc. Most of these techniques however cater to a specific application or environment. The need of the day is an interoperable and standardized generic system that can greatly reduce the false alarm rate.
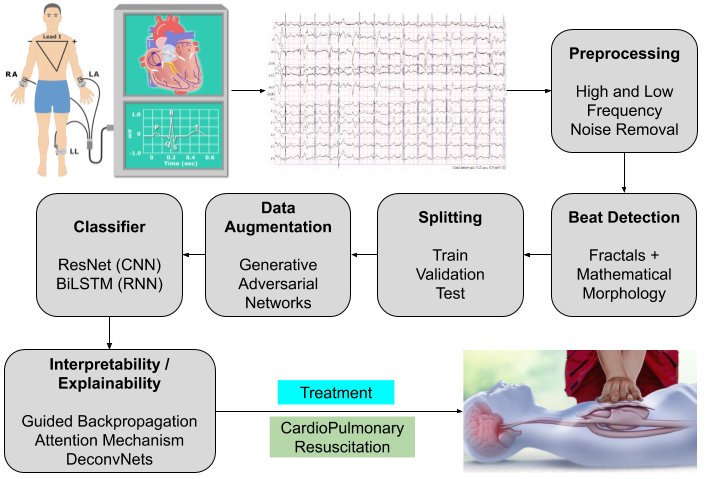
An Interpretable Machine Learning Framework using Deep Learning Models for the Classification of Electrocardiogram Signals
Healthcare is now necessary more than ever in the times of COVID-19. It enhances the quality of life, along with the prevention of premature death and disability. Our goal encompasses disease prevention and forestalling death by prognosis of disease.Deep learning models are used as these models are becoming ubiquitous in the Healthcare domain and have seen rapid development in recent years. The real-world use case of these models is limited due to the latent reasoning behind these models’ decisions. Therefore, we employ various interpretability phenomena to provide visual explanations for the model predictions. Our work focuses on arrhythmia detection using electrocardiogram signals acquired by non-invasive tests.